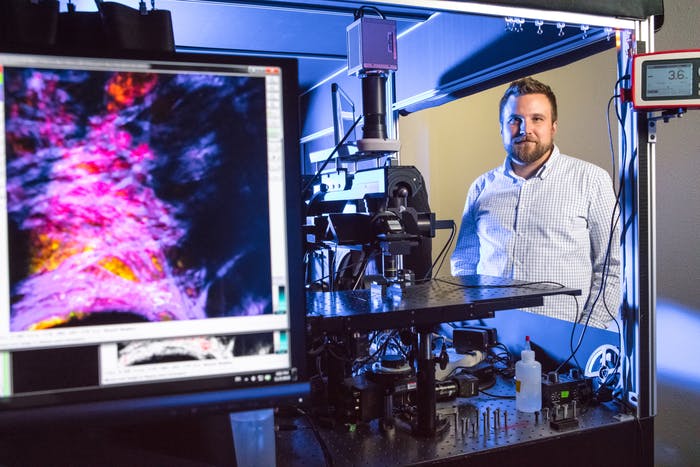
University of Arkansas biomedical engineering professor Kyle Quinn received a four-year, $1.6 million grant from the National Institutes of Health to develop non-invasive, real-time “optical biopsies” of chronic skin wounds.
我们的目标是provide digital histopathology images and other quantitative information without the need for an invasive biopsy, tissue processing and staining with histology dyes.
The initial clinical assessment of a chronic skin wound involves visual inspection, but more detailed characterization relies on histological analysis of wound tissue biopsies. While this approach is useful in clinics and research labs for understanding wound pathophysiology and developing new products to treat chronic skin wounds, it is inherently invasive, time-consuming and qualitative.
For several years, Quinn has been working on an alternative, quantitative imaging system that addresses some limitations of conventional histological analysis. Researchers in his lab use multiphoton microscopy to view tissue in three dimensions at the cellular level and generate 3D maps of wound metabolism. This imaging technique is non-invasive, which allows them to measure changes in cellular metabolism and skin organization over time within the same wounds.
Although their metabolic imaging technique can provide highly detailed assessments of cellular function, the analysis of their image data takes time. Researchers must manually map relevant image regions to specific layers of the skin or wound regions, which is a slow and tedious process. To speed it up, Quinn has partnered with Justin Zhan, professor of computer science and computer engineering. Zhan, a data science expert, is helping Quinn combine multiphoton microscopy and “deep learning,” an artificial intelligence-based approach to analysis.
The deep learning approach will enable Quinn to provide rapid quantitative analysis of chronic skin wounds.
“Through deep learning we can train a computer algorithm to delineate wound regions accurately and very quickly,” Quinn says in a news release. “This will greatly speed up our analysis and remove the subjectivity and bias that is inherent when you ask humans to assess images and identify features.”
Preliminary results on the use of deep learning to identify wound features appear in theJournal of Investigative DermatologyandLasers in Surgery and Medicine.
In addition to Zhan, Quinn will collaborate with leaders in the wound healing field, including Aristidis Veves, research director of the Joslin-Beth Israel Deaconess Foot Center, and Marjana Tomic Canic, professor of dermatology at the University of Miami. By combining wound image data from multiple labs, the team will have a more diverse set of data to rigorously train neural networks that can broadly work for different kinds of wounds.